Strategies based on social network analysis for enhancing the learning climate at universities
Abstract
The aim of this study was to detect leaders among a group of university students based on their centrality scores and, from these scores, to distribute roles to help enhance learning climate. We understood that learning climate improved when the construction of networked knowledge was stimulated from the perspective of Computer-Supported Collaborative Learning. We conducted the educational experiment in a virtual environment. An online discussion forum was set up in a loosely structured format, and the students’ centrality scores were calculated from the social network they generated in the forum. Our findings show that student connectivity increased significantly and that several leadership styles were detected. Based on these leadership styles we designed strategies for optimizing learning climate in a self-regulated and stable way. Based on the type of centrality, we detected leaders in terms of their popularity, sociability, closeness to others, control of information flowing through the network, and influence. The novelty of this study resides in the incipient production of educational technology based on Social Network Analysis and, specifically, on the design of centrality-based strategies for optimizing climate in the university classroom.
Keywords
social networks, online discussion, educational technology, leadership, higher education
Resumen
El objetivo de este estudio fue detectar líderes a partir de los puntajes de centralidad de un grupo de estudiantes universitarios para repartir funciones que contribuyeran a optimizar el clima del aula. Entendimos que el clima mejoraba estimulando la construcción de conocimiento en red desde un entorno de aprendizaje colaborativo con apoyo de computadoras. La experiencia educativa se llevó a cabo en un entorno virtual. Se configuró un foro de discusión en línea para los estudiantes con formato loosely-structured y se calcularon los puntajes de centralidad a partir de la red social que generaron en el foro. Los resultados muestran un crecimiento significativo en la conectividad de los estudiantes y se detectaron diferentes estilos de liderazgo, en función de los cuales diseñamos estrategias para optimizar el clima del aula de manera autorregulada y estable. Dependiendo del tipo de centralidad, se detectaron líderes en popularidad, en sociabilidad, en cercanía a los demás, en control de la información que fluye a través de la red y líderes en influencia. La novedad del estudio consiste en la producción incipiente de tecnología educativa basada en Análisis de Redes Sociales, y concretamente el diseño de estrategias basadas en la centralidad para optimizar el clima de un aula universitaria.
Palabras clave
redes sociales, discusión en línea, tecnología educativa, liderazgo, educación superior
Resum
L’objectiu d’aquest estudi va ser detectar líders a partir de les puntuacions de centralitat d’un grup d’estudiants universitaris per repartir funcions que contribuïren a optimitzar el clima de l’aula. Vam entendre que el clima millorava estimulant la construcció de coneixement en xarxa des d’un entorn d’aprenentatge col·laboratiu amb suport d’ordinadors. La experiència educativa es va dur a terme en un entorn virtual. Es va configurar un fòrum de discussió en línia per als estudiants amb format loosely-structured i es calcularen les puntuacions de centralitat a partir de la xarxa social que generaren en el fòrum. Els resultats mostren un creixement significatiu en la connectivitat dels estudiants i es van detectar diferents estils de lideratge, en funció dels quals dissenyarem estratègies per optimitzar el clima de l’aula de manera autoregulada i estable. Depenent del tipus de centralitat, es detectaren líders en popularitat, en sociabilitat, en proximitat, en control de la informació que flueix a través de la xarxa i líders en influència. La novetat de l’estudi consisteix en la producció incipient de tecnologia educativa basada en l’Anàlisi de Xarxes Socials, i concretament el disseny d’estratègies basades en la centralitat per millorar el clima d’un aula universitària.
Paraules Clau
xarxes socials, discussió en línia, tecnologia educativa, lideratge, educació superior
Practitioner notes
What is already known about the topic
-
Social Network Analysis (SNA) detects learning communities in Higher Education where students build networked knowledge on specific issues.
-
Online Discussion Forums (ODF) are social networks where students' knowledge building increases as they post more in the forums.
-
Published papers report no empirical evidence to use centrality-based educational technology to help university students to build networked knowledge from leadership.
What this paper adds
-
We provide a method to detect leaders in the classroom based on students' centrality scores. The scores help to design teaching strategies to optimize the climate for networked knowledge construction.
-
The novelty of this study is the production of educational technology based on Social Network Analysis, although this research line is still under development.
Implications of this research and/ or practice
-
The centrality-based strategies we propose here are feasible as students' connectivity grew significantly along the training period.
-
More empirical evidence is necessary to obtain the functionality of these strategies for networked knowledge building. However, the method we provide lets us design them.
Introduction
Educational technology based on Social Network Analysis (SNA) includes centrality measures, which detect different leadership styles. When that network represents a university classroom, teachers can define strategies based on student centrality to optimize the classroom climate by involving several key individuals. One of the most recurrent instruments to extract data and conduct SNA is the Online Discussion Forum (ODF) (Saqr, Viberg, & Vartiainen, 2020; Silva, Barbosa, & Gomes, 2019; Zou et al., 2021). According to recent findings, asynchronous online discussion favors university students’ participation (Chen & Liu, 2020) and collaborative knowledge building (Heijst, Jong, Aalst, Hoog, & Kirschner, 2019). That can be a helpful strategy to project their class participation and foster an appropriate learning climate, taking advantage of an analyzable data set.
Classroom climate includes the mood, attitudes, and rules that people share in the classroom. We aimed to strive for an interactive and inclusive environment through SNA, where students were not so concerned about grades but about their learning process.
Many of the studies in this regard relied on constructivist pedagogy and a social-cognitive approach (Dommett, 2018). Therefore, the overall purpose of using ODF has been to produce an atmosphere that stimulates networked knowledge construction. This atmosphere can be stabilized in a self-regulated way over a certain training period, following models of co-regulation of learning (Hadwin, Järvelä, & Miller, 2018). Nevertheless, the effect of social regulation on e-learning is still under evaluation (Hwang, Wang, & Lai, 2021). In this line, centrality metrics provide reasonable criteria for managing learning in a university classroom through networked knowledge construction.
The purpose of this study was to detect different types of leaders using students' centrality scores to assign them with leadership roles that contribute to optimizing the classroom climate. We contrasted the hypothesis that the learning climate enhances as the construction of networked knowledge is stimulated in computer-supported collaborative learning environments. The study had an exploratory approach since we looked for empirical evidence after piloting a teaching innovation activity. Most published studies about ODF with SNA at the university level aimed to establish a theoretical framework for the analysis itself (Jan & Vlachopoulos, 2019) or focused on detecting learning networks (Jan, 2018). However, we did not find as many studies on SNA-based educational techniques to stimulate learning. The novelty and relevance of our experience rely precisely on the production of SNA-based educational technology, although this research line is still under development.
We did not conduct the ODF to obtain evidence of validity and reliability for any teaching method. It was a teaching innovation activity, which still needs empirical evidence to support its effectiveness. With this in mind, we designed teaching strategies to improve the classroom climate in a self-regulated way based on centrality metrics extracted from the forum postings.
Method
This paper reports a pilot activity designed to assess the centrality of an undergraduate student group. The exercise consisted of an ODF that let us collect data for SNA, following recommendations in previous studies (Lucas, Gunawardena, & Moreira, 2014). Moreover, the use of forums is frequent in our context (Gómez-Gonzalvo & Tella-Muñoz, 2012; Martínez, 2010), and we assumed the study as a contribution to the development of teaching in universities from our region. The ODF lasted for ten weeks.
Participants and learning environment
The activity took place at the University of Valencia with a group of first-year undergraduate students enrolled in Social Education degree. We set up an ODF to assess their involvement in the compulsory subject of Social Pedagogy. According to the syllabus, the subject assessment consisted of three blocks. The students handed in a portfolio with theory activities worth 50% of the total score, 30% depended on a team project, and 20% on participation during the course. However, it was not clear how to measure participation, even though it was a high percentage. Thus, we thought that students should participate beyond class attendance and classroom interventions, so we created a forum for them to interact and learn online and asynchronously.
This group was composed of 50 students, including 39 females and 11 males. Although irregular participation could have hampered to reach of firm conclusions, eventually, experimental mortality during the educational experience was not a reason to cancel the trial. Once we completed the activity, we had to exclude three female students from the sample because their participation had been too low and distorted the analysis of results. The group we finally analyzed included 47 students plus the teacher since he participated in the activity with only one introductory post in the forum. This post served as an example and opened up a topic for discussion, inquiring the students whether they considered this subject was helpful for their training and why.
The students had to read the comments posted in the ODF and answer to their classmates to establish a peer-to-peer dialogue and build or "negotiate" (Fabbri, 2018) networked knowledge. In this sense, the ODF constituted a directed learning network where one student would allude to another regarding his or her comments, without the latter necessarily giving a response or interacting with the former.
Task objectives
The activity had three main objectives: (1) learning about social pedagogy and (2) about classmates, and (3) enhancing the training process of the class group. We defined these objectives as follows, respectively.
-
Students build their knowledge related to social pedagogy asynchronously while interacting with each other in an online collaborative learning environment. We understood interaction is a necessary factor for networked knowledge building.
-
They become better acquainted with the cognitive and professional interests of the rest of their peers, establishing links with as many people as possible.
-
The teacher disposes of centrality metrics that help to optimize the students' training experience.
Our study focuses mainly on the third objective because it is the only one that we could not contrast with other activities that offered evidence of student involvement in the subject. We divided the goal of obtaining centrality metrics into five operational aims: detecting leaders in popularity, sociability, influence, access to peers, and controlling information flows in the ODF. A detailed definition of leadership styles lay along sections 2.5 and 3.1. The leader detection system facilitated the production of teaching strategies to improve classroom climate and encourage self-regulation.
Settings of the learning management system
At the University of Valencia, we regularly work with Moodle (https://moodle.org/). In the learning management system, we organize the subjects and courses where we manage our teaching activities. Thus, we set up the ODF on this platform the institution provided.
During the configuration phase, we looked for an appealing title to attract student participation. We added a brief description of the task objective, and we did not restrict student access to the forum at any time. We also opted for a loosely structured format (Dommett, 2018), slightly more structured than envisaged in traditional classifications (Hammond, 2005). Below we indicate the rules of participation that define the structure we designed for the activity.
When we set up the ODF, we thought it would be a good idea for students to upload files to their posts. So, they could provide evidence for their arguments, materials for further information, or other similar resources. However, no one uploaded files. Some students added images directly to their posts, along with the text, and others included URLs to web pages with supplementary information. Either way, we enabled the file upload option, and it remained enabled throughout the course.
We use the Urkund automatic text recognition system (https://www.urkund.com/) to detect the amount of plagiarism in each post. We penalized plagiarism of more than 15% by reducing the mark for this part of the assessment in the subject. The penalty was progressive. The higher the plagiarism, the higher the penalty. It was the only assessment-related system we set up in the ODF. We did not use any system on the platform to grade the students, as these were marks based on reductions, such as the one applied for plagiarism, and it was easier to assess them using a Microsoft Excel spreadsheet.
We fed the connections between students into specialized software to obtain SNA-related aspects of the assessment. In this case, we used Gephi 0.9.2 (https://gephi.org/), as the Moodle features did not include the possibility to assess students with SNA-based parameters. We could have installed some plug-ins, but the available ones did not offer the functions we needed to calculate student centrality.
Guidelines for participation in the network
While designing the activity, we established some guidelines for participation in the ODF. The purpose of following the rules was to facilitate the teacher's assessment and the students' management of the platform. We tried to reduce the number of rules as much as possible and assigned a name to each one to memorize them better. The guidelines were as follows.
-
Title. Students assigned a heading to each post to quickly identify its content.
-
Q/A. Students distinguished the posts as either questions or answers, and never both at the same time.
-
Direction. Students cited previous posts when publishing on the ODF to ask or answer questions related to the discussion thread.
-
Posting. Students posted at least once every week until the end of the semester.
-
Peer-assessment. Students indicated the usefulness of each post they cited on a scale from 1 to 3. That allowed to assign weights to the edges in the learning network.
-
No changes. We restricted post modifications after publishing.
According to previous research, when students can find and select the information they need, and especially when they have prompts in the ODF, they tend to read and reply to their peers more frequently (Wang & Yang, 2012). We did not activate prompts because they might reduce the cognitive load that students can bear (Sachdeva & Gilbert, 2020), hindering their attention span and favoring an apathetic climate in the group. Instead, we recommended using the search engine to filter the posts.
In Moodle, the search engine did not include post titles, so we recommended writing the words of the title in the body text and provide short and informative headings. We found this helpful to find the information they needed, and they could focus the activity on their learning interest.
Student knew whether the information they provided in the ODF was relevant to the group because their classmates rated it. However, we did not consider peer assessment for grading in any circumstances and made this clear in the classroom several times since the beginning.
Although the aim was not to detect Frequently Asked Questions (FAQ), we based on the Question Answering Forum model (Sindhgatta, Marvaniya, Dhamecha, & Sengupta, 2017) because the questions foster knowledge building (Gargallo-López, 2017). Therefore, we managed a learning network for students to address questions to each other.
We decided to forbid post-editing once published because of the need for monitoring the progress of each student.
In the beginning, it was a struggle to get the students to follow all the rules, but they soon learned and respected them.
Assessment of centrality
In SNA, the centrality of a node is equivalent to its relative importance within a network of which that node is an integral part. SNA provides a framework for analyzing learning and participation at the university (Jan et al., 2019), especially in students' networked inquiry into new knowledge issues (Jan, 2018). Here, the network nodes represented the students. When they inquired about each other's input in the ODF, they created a network where it was possible to identify to what extent one person had more influence than another or the authority they acquired within that network. We obtained degree, eigenvector, closeness, and betweenness centralities of each student from the ODF to determine their importance in the learning network.
The simplest way to obtain centrality was to sum the number of links a node v ∈ V had (Sun & Tang, 2011), where v was the node and V was the set of nodes that made up the network. These links were the degrees that each node had. In our directed network, there were in-degrees when the node was the final vertex of the link or edge, and there were out-degrees when the node was the initial vertex. We estimated centrality from the in-degrees to obtain popularity scores and from the out-degrees to find the students' sociability in the ODF context. An example outside the forum may help to clarify this idea.
In the directed network of Figure 1, the node with the highest degree centrality is v1 because it has more edges ei than the others. However, the most popular is v4 because it has twice as many in-degrees as any other node in the network. The node v1 is also the most outgoing, as it has produced three times as many out-degrees as any other node, while v4 is among the least sociable, despite being the most popular.
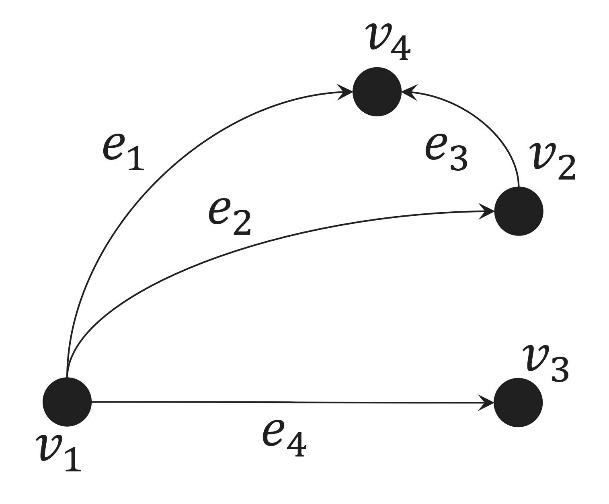
We obtained the eigenvector centrality to know the influence of each student in the ODF. We first estimated the product of Ax, where A is the adjacency matrix, and x is the vector resulting from the degree centralities of the nodes directly connected to a given node. Thus, the sum of these centralities gave a value for each node, providing the basis for contrasting the eigenvector centrality that Gephi reported. Those nodes with higher eigenvector centrality connected to other nodes that also had a high level of connection, linking several groups of students, which at the same time formed clusters in the network.
We calculated the students' closeness centrality (i.e., the shortest distance in the network from each of them to all the others) (Freeman, 1979) based on the classical work by Sabidussi (1966). This score provided information about their accessibility to each other in the ODF. The computational function consisted of summing the vectors of the distance matrix i,j, considering the shortest distance between node i and node j. Therefore, we obtained the centrality for each node i by computing the reciprocal value of the sum of the distance matrix, whose i-th component was equal to 1, and the others were equal to 0. Finally, we normalized the closeness centrality in the range 0-1. Knowing the students' closeness allowed us to know who was closer to the center of the network and, therefore, speeded up the propagation of information among their classmates.
In Figure 2, node v1 has the highest centrality, and it is easier for this node to transmit information to the whole network, as there is only one degree of separation from the others. In contrast, the other nodes must take twice as many steps to spread information throughout the network, as they must pass through node v1 and then move to a third node.
Betweenness centrality was helpful to detect students who were a bridge between other students in the ODF (Figure 2). Node v1 is a bridge between all the others and acts as a mediator between them. Therefore, it is a point for information control that transmits data from one node to another. Betweenness let us know how often a student bridged along the shortest path between two other students, following Freeman (1977) notion. We normalized the scores and used theBrandes (2001) algorithm to obtain the results.
Each of these centrality metrics helped to detect different types of leaders, as shown in Figure 3. When piloting this activity, we expected to find students with diverse leadership styles. That would allow us to assign different roles to leaders and share the effort to improve the learning climate.
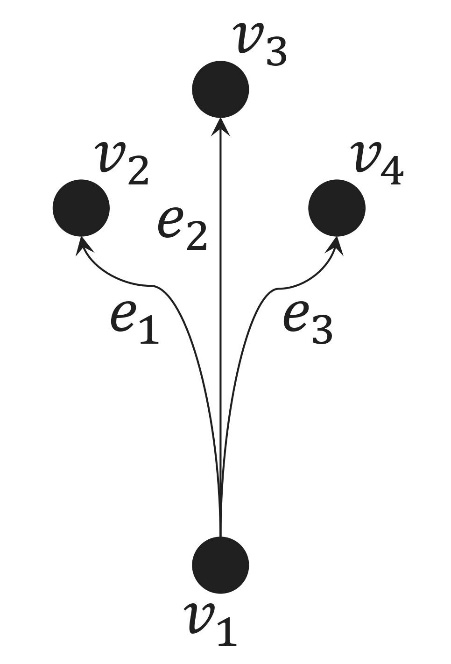
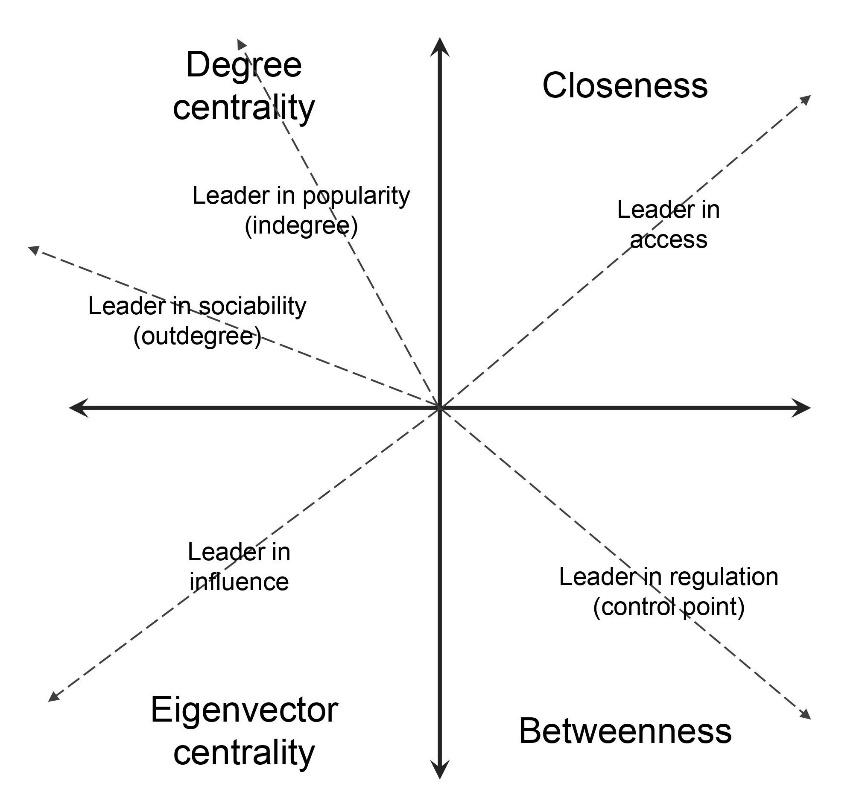
Results
The number of posts in the ODF increased steadily over ten weeks of training (Figure 4). Each post generated one or more connections among the students, although they did not post much more than requested for completing the task.
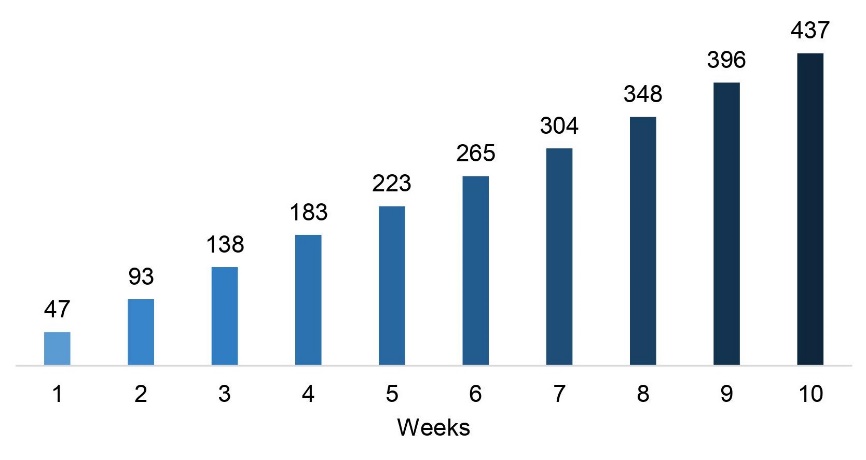
Consequently, the learning network grew until it reached a density equal to .235 (Figure 5). The evolution of the degree of connection between the students was significant week after week until the training concluded (Table 1).
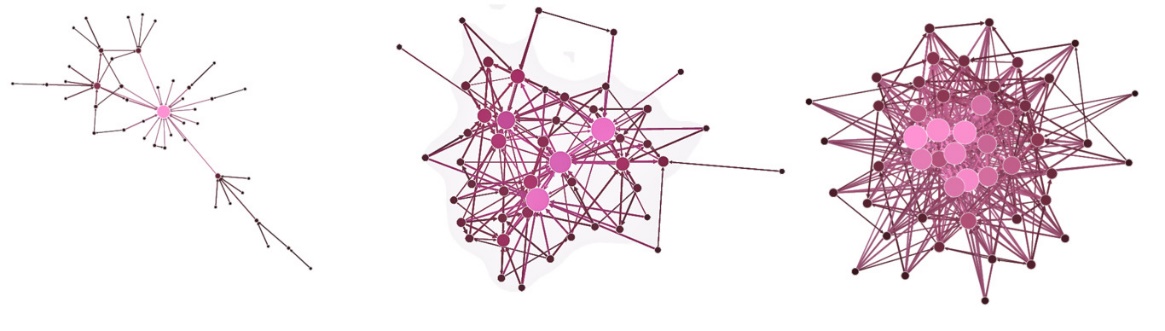
Week (W) |
W1 |
W2 |
W3 |
W4 |
W5 |
W6 |
W7 |
W8 |
W9 |
W10 |
---|---|---|---|---|---|---|---|---|---|---|
N |
49 |
49 |
49 |
49 |
49 |
49 |
49 |
49 |
49 |
49 |
Mean |
2.12 |
4.33 |
7.02 |
9.02 |
10.61 |
12.73 |
14.61 |
17.10 |
19.84 |
21.92 |
Median |
1.00 |
3.00 |
5.00 |
6.00 |
8.00 |
10.00 |
11.00 |
13.00 |
15.00 |
16.00 |
IQR* |
1.00 |
2.00 |
5.00 |
7.00 |
8.00 |
10.00 |
11.50 |
16.50 |
19.50 |
21.50 |
Contrast |
W1-10 |
W2-10 |
W3-10 |
W4-10 |
W5-10 |
W6-10 |
W7-10 |
W8-10 |
W9-10 |
|
Z** |
-6.095 |
-6.094 |
-6.095 |
-6.096 |
-6.099 |
-6.100 |
-6.104 |
-6.113 |
-5.749 |
|
Two-tailed p |
.000 |
.000 |
.000 |
.000 |
.000 |
.000 |
.000 |
.000 |
.000 |
|
Finally, we detected different types of leaders to whom we assigned different roles to optimize the learning climate. Table 2 shows the ten students with the highest centrality scores after the last week. Student 33 was the only one who accumulated two different leadership types.
Participants |
Indegree* |
Outdegree* |
Closeness centrality |
Betweenness centrality |
Eigenvector centrality |
Leadership style |
---|---|---|---|---|---|---|
Student 3 |
85 |
43 |
0.58 |
0.08 |
0.68 |
Access |
Student 8 |
94 |
34 |
0.51 |
0.04 |
0.62 |
|
Student 13 |
74 |
61 |
0.56 |
0.06 |
0.57 |
Sociability |
Student 33 |
129 |
25 |
0.47 |
0.09 |
0.72 |
Popular/Control |
Student 34 |
18 |
26 |
0.51 |
0.06 |
0.22 |
|
Student 40 |
70 |
43 |
0.55 |
0.04 |
0.51 |
|
Student 41 |
80 |
26 |
0.46 |
0.01 |
0.77 |
Influence |
Student 47 |
79 |
54 |
0.55 |
0.05 |
0.77 |
Influence |
Student 49 |
79 |
42 |
0.53 |
0.05 |
0.57 |
|
Student 50 |
1 |
36 |
0.55 |
0.02 |
0.02 |
|
Teacher 51 |
82 |
0 |
0.00 |
0.00 |
1.00 |
|
Centrality-based strategies
From the third week onwards, it was possible to assign leadership roles and guide the leaders' activities with the other classmates. Generally, we chose only one leader for each leadership style determined by the centrality scores of the students. As the weeks went on, the ODF progressed. Sometimes, we replaced a particular leader because another student had scored higher in the type of centrality that gave him or her that leadership style.
Leaders in popularity acted as a model for their peers. They received a tutorial on the modeling technique based on Bandura (1986) social-cognitive approach, which contrasts with behaviorism. The purpose for these students was to understand the difference between imitative learning and behavioral conditioning to enable them to be a learning stimulus for others, besides the influence of final grades in student's motivation. We tried to foster the internal motivation to learn, contributing to a more formative learning climate rather than focusing on the final scores.
One of the features of leaders in sociability was to post information more frequently than other students. The role of these leaders was to stimulate passive people in the network, replying to their posts and helping them have more presence in building the discussion thread. We also contacted these leaders for a tutorial to receive supervision to activate certain people in the network, contributing to a more interactive learning climate.
Although there was a leader in popularity, not all students learned by imitation. It was often the case of students on the periphery of the network, who had few connections compared to the others. The role of the leaders in access was to help these students to increase their connectivity. The teacher guided these leaders to help create a more inclusive learning climate.
The leaders in regulation established bridges between several pairs of students who had no direct contact with each other. They played an intermediary role and could produce such connexion between these pairs of nodes, fostering transitivity in the network. Therefore, they received a tutorial where the teacher explained the concept of transitivity and gave them a list of people to contact in the ODF. In this way, the leaders in regulation generated a learning climate where information flowed more quickly among students and promoted team knowledge building and group cohesion.
The leaders in influence also encouraged group cohesion. Compared to the leaders in regulation, they worked as a bridge between pairs of clusters and not only between pairs of nodes. Their purpose was to disseminate relevant information among the leaders in each clique to optimize social knowledge building and generate a learning climate with a higher level of updating on new developments and changes in the course.
Leadership roles were rotating during training weeks, and we tried to get several students to learn to lead in different ways according to their centrality scores. When another student exceeded the centrality score, we thanked an active leader for collaborating, and we asked him or her to stop exercising this role so that others could learn to exercise it as well.
Discussion and conclusions
Self-regulation of the learning climate
The co-regulated learning models (Hadwin et al., 2018) generally refer to small groups of students (Järvelä, Järvenoja, Malmberg, & Hadwin, 2013), and the ODF complimented them for larger groups. However, we need to obtain more empirical evidence based on centrality scores to define the role of students who work in the same team. After piloting this activity, we concluded that centrality-based strategies could help optimize the learning climate, generating coherent and self-regulated networked knowledge building. Therefore, we consider the ODF to complement other activities that we usually propose in class, such as team projects with few students in each team.
The centrality scores provided some criteria for teachers to select several student delegates in the same group. They would exercise different functions in the group to optimize the learning climate. The sharing and rotation of leadership roles facilitated a dynamic learning climate for networked knowledge management and construction, not just online but also face-to-face. At least, the results suggested so in terms of students' connectivity (Table 1) and their leadership capital (Table 2).
Other experiences of educational innovation
Other similar experiences focused on student grades, giving more weight to outcomes compared to the learning process (Alzahrani, 2017). Here, our interest was the process and the learning climate of the classroom during the semester. Previous studies advanced on topic classification and modeling (Zhao, Jiang, & Gray, 2019), discourse analysis, and the students' interaction for learning (Onyema, Deborah, Alsayed, Noorulhasan, & Sanober, 2019; Wang et al., 2019). That included formative assessments but was more in line with higher-order cognitive skills. Our experience was more focused on leadership and social dynamics, which could improve the learning climate.
In previous experiences with ODF to train students in leadership skills (Bleich, 2020), leadership was not assessed through SNA, as we did. In contrast, when conducting SNA, it was mainly in forums of Massive Open Online Courses (MOOCs) (Amastini, Kaunang, Nefiratika, Sensuse, & Lusa, 2020; Zou et al., 2021). Our study consisted of a trial with first-year undergraduate students, and the educational implications could be different due to the knowledge requirements of each context. While undergraduate students try to learn the basics of an academic discipline, MOOCs usually train students to delve into learning content previously acquired.
We have not yet found other teaching experiences where SNA was used in the ODF to detect leadership styles and guide students towards improving the learning climate.
Limitations and emerging research
Although we achieved the aims of the pilot trial, we found limitations with the ODF. Students did not always respect the guidelines, increasing the risk of systematic errors in the data analysis. Moreover, the ODF partially reflected the learning climate, so the findings offer an incomplete perspective on the circumstances in class. The forum generated connections between people, who probably continued to connect outside of this activity, but at the very least, it provided social contact. They achieved a greater awareness of the rest of their classmates. In contrast, traditional teamwork would have only allowed a limited number of people in the class to interact in several isolated closed networks.
We understand this is a complex procedure, and not all university teachers know the requirements of SNA and software management to compute centrality scores. However, this does not restrain the exploration of centrality-based teaching strategies. Contrary, the evidence from this experience is a reason to find resources to facilitate using these strategies. Meanwhile, more empirical evidence is necessary to strengthen our knowledge about the effectiveness of these strategies and outline their usefulness in different scenarios.